How to build a data governance framework to realise the value of AI in Australia
Implement robust data governance for AI success in Australia. Ensure data security, quality, and compliance with effective strategies and practical examples.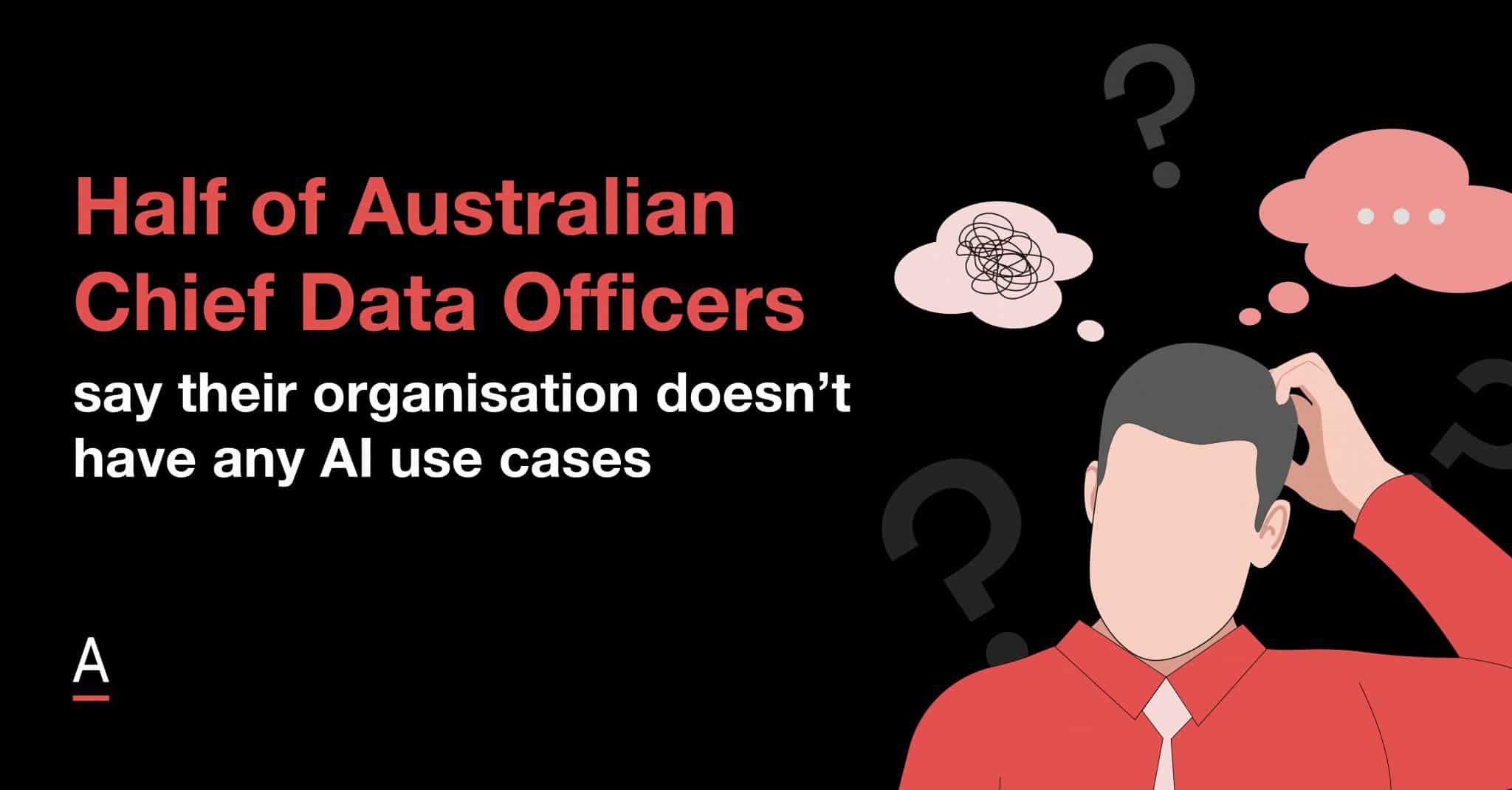
Effective data governance is crucial for the successful implementation of AI, and organisations without comprehensive data governance face several challenges.
Data security issues are a primary concern.
The absence of robust governance frameworks often increases breaches and unauthorised access risks.
Organisations are more vulnerable to data leaks and cyber-attacks without structured access controls and monitoring, which can result in severe financial and reputational damage.
Data quality problems are also prevalent when governance is lacking.
Inaccuracies, inconsistencies, and duplicate data undermine the reliability of AI models.
Flawed data leads to unreliable and potentially harmful decisions.
Compliance challenges are another issue.
Organisations without solid data governance frameworks need to work on compliance with data protection regulations like GDPR and CCPA, which can lead to substantial fines and legal consequences.
Managing audit trails, ensuring proper documentation, and maintaining compliance is difficult without a structured approach.
These challenges highlight the need for robust data governance, not just for managing and protecting data effectively, but also for ensuring the success of AI initiatives.
5 Steps to Build Data Governance
Building a robust data governance framework involves several critical steps to ensure data is managed effectively, securely, and in compliance with regulatory requirements.
Step 1: Assess the Current Data Landscape
Conduct a comprehensive evaluation of the organisation’s existing data landscape. This involves:
- Identifying different data types
- Pinpointing critical data assets
- Measuring data quality
- Evaluating current data access protocols
This assessment provides a foundational understanding of the existing conditions and highlights areas needing improvement, forming a baseline for developing a governance strategy.
Notably, 94% of organisations focus on providing accurate data for business decision-making, according to ADAPT’s 2023 Data Edge Survey of 92 Australian data leaders.
In a recent panel discussion at the Data & AI Edge conference, data executives Dayle Stevens, Executive – Data & AI at Telstra, Claudine Ogilvie, CEO at HivePix, and Joanna Gurry, Executive – Data Platforms at NAB shared their insights on mastering data maturity and harnessing AI to create value and drive business success.
The conversation revolved around organisational maturity, data governance, and leveraging AI to solve complex business problems.
Joanna highlighted the importance of understanding the implications of data quality, security, and privacy in data governance, advocating for boldness and patience in driving organisational change.
She shared examples of how external pressures can trigger the adoption of data governance, ultimately enhancing data quality and consistency.
Dayle discussed the challenge of understanding and managing the vast data landscape within large organisations, advocating for simplification to improve data management and protection.
She emphasised the importance of involving everyone in data governance and building trust in data, regardless of its purpose.
Step 2: Develop and Implement the Data Governance Strategy
Actively involve various stakeholders, including IT, security, business teams, and compliance officers.
Clear roles and responsibilities are crucial to ensure accountability and effective execution.
Collaboration among stakeholders is essential for addressing the multifaceted challenges of data governance.
With 92% of organisations focusing on compliance with data security, privacy, and regulatory regulations, this step is critical.
Similarly, Dhivian Govender, Head of Digital Data & Analytics at Slater & Gordon Lawyers, shared his insights on addressing data challenges in the legal sector during an interview at the Data & AI Edge conference.
Dhivian emphasised the importance of data quality in the legal industry, which handles both structured and unstructured data.
He advocated for collaboration between data practitioners and end-users to define and address data quality issues related to critical data assets.
Additionally, he stressed the need for a balance between data-driven customer experiences and privacy, calling for strong data usage policies and robust governance frameworks.
Step 3: Optimise Information Architecture Documentation
Map out how data flows within the organisation, including identifying data sources, processing pathways, and storage locations.
Decide on a centralised, decentralised, or distributed data governance model based on the organisation’s specific needs and risk profile.
Accurate documentation helps understand data interactions and dependencies, which is essential for informed decision-making.
As 74% of organisations aim to enhance customer experience, the chosen model should facilitate seamless data flow to support customer interactions.
Step 4: Evaluate and Enhance IT Tools and Processes
Assess the effectiveness of current IT tools and processes in supporting data management and governance. Consider how well these tools facilitate data digitisation, management, and security.
A security-by-design approach is recommended to protect data processes from inception, maintaining data integrity and confidentiality.
This involves critically evaluating existing tools and the potential need for new investments. Streamlining processes is also a priority, with 67% of organisations focusing on enhancing efficiency.
Step 5: Adopt an Agile, Iterative Governance Model
Due to the dynamic nature of data environments, adopt an agile and iterative approach.
A flexible governance model allows for regular assessments and updates to governance practices, helping the organisation adapt to new challenges and opportunities.
This continuous improvement mechanism ensures that the governance model remains relevant and practical.
Since 65% of organisations aim to fuel revenue growth through effective data governance, maintaining an adaptable framework is essential.
Components and Building Blocks of Data Governance
ADAPT laid a framework that outlines the key building blocks necessary for a robust data governance strategy.
Ownership
Ownership involves establishing roles such as data owners and data stewards, which improves accountability and responsibility for data efforts.
Secure agreement on a shared data vision and continuous sponsorship from the business.
User-Centred Approach
Adopting a user-centred approach means incorporating a common language and including users across the business at the centre of data sharing and access controls.
Ensure users are actively involved in the design and implementation of data governance policies to build data literacy and user trust.
Security by Design
Security by design focuses on implementing data access controls, risk detection, and mitigation strategies to reduce risk and enhance security.
Develop and enforce comprehensive security policies and incident recovery management plans.
Metadata Management
Effective metadata management involves setting up data catalogues and standardising data collection, storage, and quality management.
Create and maintain detailed data catalogues and ensure standardisation across the organisation to establish a solid foundation for data use and future transformation, such as AI model development.
Policies and Regulations
Establishing robust policies and regulations is key to ensuring safe and ethical data use while reducing risk.
Develop clear data policies that align with regulatory requirements such as GDPR and APPs and set up data access controls.
Monitor and Pivot
Monitoring and pivoting involve setting up key performance indicators (KPIs) to regularly monitor and audit data governance efforts.
Regularly review data processes, measure their effectiveness, and make adjustments as needed to maintain data quality and integrity.
By integrating these components into a cohesive strategy, organisations can establish a robust data governance framework that supports their business goals and ensures compliance with regulatory requirements.
Australian organisations leading the way in data governance excellence
Scentre Group Improves Real-Time Insights and Efficiency
Scentre Group, a player in the retail real estate sector, embarked on a data governance journey under Prabu Ruben, Senior Manager of Data Engineering, Integration, and Analytics.
They faced challenges with data homogeneity and the need for real-time insights, prompting the development of a unified data platform.
This platform employed a dual-cloud approach with Google Cloud Platform and Microsoft Azure for enhanced scalability and simplified, automated data retention policies to tackle data homogeneity.
A Data-as-a-Service culture was fostered, resulting in substantial financial savings.
This transformative approach led to a connected customer experience based on a single view of the customer journey, enhancing operational efficiency and enabling direct consumer engagement.
Prabu noted, “A well-governed unified platform is critical for us to create an integrated view of the customer.”
SA Power Networks Enhances Data Governance for Renewable Energy Integration
Under the guidance of Enterprise Architecture and Data Manager Matthew Pritchard, SA Power Networks has tackled the challenges posed by the rapid uptake of renewable energy.
Initially designed for traditional energy flows, the grid now needs to accommodate electric vehicles and batteries, requiring a comprehensive technology redesign to maintain safety, quality, and reliability.
To address these challenges, SA Power Networks leveraged data governance to make precise predictions about asset failures and optimise the use of renewable energy.
By enhancing data visibility and accountability by identifying data owners, users, and domains and involving them in a collaborative data council, the organisation maximised integrating green energy into the grid, avoiding unnecessary constraints and efficiently using existing infrastructure.
Matthew also highlighted the necessity of a cultural shift towards robust data literacy and governance within the organisation.
This collaborative approach has bridged silos and fostered a culture of trust and understanding around data.
For SA Power Networks, AI has become a transformative tool that elevated field operations, enhance safety, and improve efficiency by automating routine tasks, allowing workers to focus on high-value activities.
Utilities Organisation Advances Data Quality and Democratisation
AusNet Services, managing an electricity transmission network, recognised the need for a robust data governance strategy to support its transition to renewable energy.
Under the leadership of the Head of Strategy and Architecture, they spearheaded a data integration initiative to break down data silos and establish a unified data platform.
The initiative fostered collaboration with stakeholders, emphasised secure and democratised data access, and involved developing tailored data governance protocols.
Challenges like adopting a common language and navigating evolving technology were met with a shift to a model that prioritised outcomes over assets.
This user-centric approach accelerated the adoption of new technologies, improved operational efficiency, and aligned data governance with the company’s strategic objectives.
NSW Government Implements Collaborative Data Governance
The NSW Government, under Chief Data Scientist Dr Ian Oppermann, enhanced its data strategy, focusing on improving decision-making and service delivery through collaborative and consistent data use across sectors.
The government applied a structured approach to data governance using four lenses: data lifecycles, data access sharing scenarios, user and holder access methods, and risk factors.
This approach allowed them to determine appropriate control environments based on data access and personal data inclusion.
Key to their strategy was promoting cross-sector collaboration, organisation-wide data sharing, and high data literacy.
The positive outcomes led to improved decision-making processes and enhanced service delivery.
The focus on collaborative data use and stringent governance practices ensured that the government could protect privacy and security while leveraging data to its fullest potential.
Elevating effective data governance enables AI-driven decision-making, operational excellence, and business growth.
By adopting these practices, organisations can enhance their data governance capabilities, safeguard their data, drive innovation, and achieve sustained success in the AI era.